Data Analysis Procedures
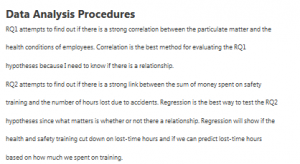
RQ1 attempts to find out if there is a strong correlation between the particulate matter and the
health conditions of employees. Correlation is the best method for evaluating the RQ1
hypotheses because I need to know if there is a relationship.
RQ2 attempts to find out if there is a strong link between the sum of money spent on safety
training and the number of hours lost due to accidents. Regression is the best way to test the RQ2
hypotheses since what matters is whether or not there a relationship. Regression will show if the
health and safety training cut down on lost-time hours and if we can predict lost-time hours
based on how much we spent on training.
RQ3 attempts to find out if we can use noise level to figure out what kind of ear protection is
best. Regression is the best way to test the RQ3 hypotheses because I need to know if there is a
relationship. Regression will demonstrate if there is a link between the use of earplugs, the
amount of noise, and hearing loss.
RQ4 is used to find out if the different training program has worked better than the old one. The t
test is the best way to find out if there is a statistically significant difference between Group A
14
and Group B employees.
RQ5 is used to identify out if lead exposure changed the amount of lead in the blood
significantly. The t test will show whether the new training program is better than the old one.
The t test is the best way to test the RQ5 hypotheses because I need to know if there is a
statistical difference between how employees were before and after they were exposed to lead.
The t test will show if the exposure caused the employees’ blood lead levels to rise.
RQ6 is used to discover out if the four lines of service all have the same average return on
investment. Since more than one line of service is of interest, ANOVA is the best way to test the
RQ6 hypothesis.
Data Analysis: Descriptive Statistics and Assumption Testing Correlation: Descriptive Statistics and Assumption Testing